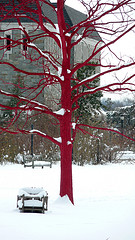
In a recent post I mentioned one of the things that amused me about Swarthmore when I first started working here. That got me to thinking about all the things that I found, then and now, to be so charming. So in this Thanksgiving season, I thought I’d share a few of them …
- Candy or snacks in all of the student services offices, as well as many academic department offices.
- The occasional frisbee flying into my office (when I was on the third floor) from the adjacent wing of Parrish – which is a men’s residence hall .
- Former Dean Bob Gross’s springer spaniel, Happy, roaming the hallways looking for the dog treats available to him in all the offices. And all the other dogs around campus – George and Ali, the bookstore dogs, Dobby, and the rest.
- Red and blue trees and the occasional tree sculpture.
- Jake Beckman’s (’04) artwork – the big chair on Parrish lawn, the giant sneakers hanging off a chimney of Parrish, and the giant lightswitch on McCabe Library.
- The tin of candy that one of my colleagues brings to meetings she attends, for sharing. Round and round the table it goes… sweet!
- The fact that so few people refer to their own titles when introducing themselves – just their office. (A little confusing at first, perhaps, but that’s alright.)
- The Swarthmore train station (regional rail) at the end of Magill walkway. In the snow. It’s like a postcard.
- The beautiful portrait (painted by Swarthmore’s Professor of Studio Art Randall Exon) in the entryway of Parrish of Gil Stott with his cello.
- Discovering the hidden talents and passions of people who work here. There are singers, actors, stargazers, songwriters, woodworkers, animal activists, knitters, world travelers – it’s amazing!
- The “honker,” which is the Swarthmore’s fire station’s version of a siren. Of course I’m not happy to think there might be a tragedy – I just enjoy its uniqueness.
- The labels on all the trees and plantings, because the College grounds are the awesomely gorgeous Scott Arboretum.
I’m sure there are many things I’ve missed. I’d love to hear about others’ favorites!